Much has been and is being said about the vagueness of the notion of “metaverse”. To compensate for this, the current trend is to add an adjective to the “metaverse” name. So, now we have studies on industrial metaverse, medical metaverse, tourist metaverse, and more.
In the early phases of its metaverse studies, when it was scoping the field, MPAI did consider 18 metaverse domains (use cases). Now, however, that phase is over because the right approach to standards is to identify what is common first and the differences (profiles) later.
In this paper we will try to identify features that are expected to be common across metaverse instances (M-Instances).
The basic metaverse features are the ability to:
- Sense U-Environments (i.e., portions of the Universe) and their elements: inanimate and animate objects, and measurable features (temperature, pressure, etc.). By animate we mean humans, animals, and machines that move such as robots.
- Create a virtual space (M-Instance) and its subsets (M-Environments).
- Populate virtual spaces with digitised objects (captured from U-Environments) and virtual objects (created in the M-Instance).
- Communicate with other M-Instances.
- Actuate U-Environments as a result of the activities taking place in the M-Instance.
How will such an M-Instance be implemented?
We assume that an M-Instance is composed of a set of processes running on a computing environment. Of course, the M-Instance could be implemented as a single process, but this is a detail. What is important is that the M-Instance implements a variety of functions. Here we assume that functions correspond to processes. These are individually activated where they are accessible at the atomic level or by a single large process.
While a process is a process is a process, it is useful to characterise some processes. The first type of process is a Device, having the task to “connect” a U-Environment with an M-Environment. We assume that to achieve a safe governance, a Device should be connected to an M-Instance under the responsibility of a human. The second type of process is the User, a process that “represents” a human in the M-Instance and acts on their behalf. The third type is a Service, a process able to perform specific functions such as creating objects. The fourth type is an App, a process running on a Device. An example of App is a User that is not executing in the metaverse platform but rather on the Device.
An M-Instance includes objects connected with a U-Instance; some objects, like digitised humans, mirror activities carried out in the Universe; activities in the M-Instance may have effects on U-Environments. There are sufficient reasons to assume that the operation of an M-Instance be governed by Rules. A reasonable application of the notion of Rules is that a human wishing to connect a Device to, or deploy Users in an M-Instance should register and provide some data.
Data required to register could be a subset of the human’s Personal Profile, Device IDs, and User IDs. There are, however, other important elements that may have to be provided for a fuller experience. One is what we call Persona, i.e., an Avatar Model that the User process can utilise to render itself. Obviously, a User can be rendered as different Personae, if the Rules so allow. A second important element is the Wallet: a registering human may decide to allow one of their Users to access a particular Wallet to carry out its economic activity in the M-Instance.
Figure 1 pictorially represents some of the points made so far.
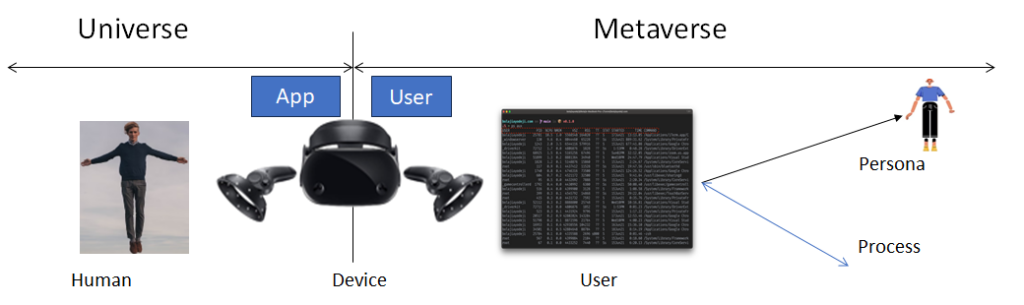
Figure 1 – Universe-Metaverse interaction
The activities of a human in a U-Environment captured by a Device may drive the activities of a User in the M-Instance. The human can let one of User:
- Just execute in the M-Instance without rendering itself.
- Render itself as an autonomously animated Persona.
- Render itself as a Persona animated by the movements of the human.
We have treated the important case of a human and their User agent. What about other objects?
Besides processes performing various functions, an M-Instance is populated by Items, i.e., Data and Metadata supported by the M-Instance and bearing an Identifier. An Item may be produced by Identifying imported Data/Metadata or internally produced by an Authoring Service. This is depicted in Figure 2 where User produces:
- Item1 by calling the Authoring Service1
- Item2 by importing data and metadata and then calling Identification Service2.
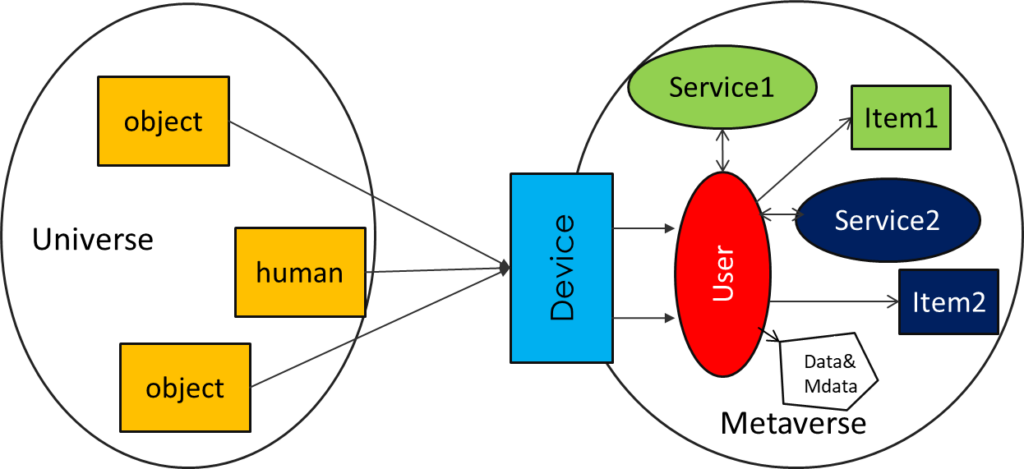
Figure 2 – Objects in an M-Instance
A more complete view of an M-Instance is provided by Figure 3.
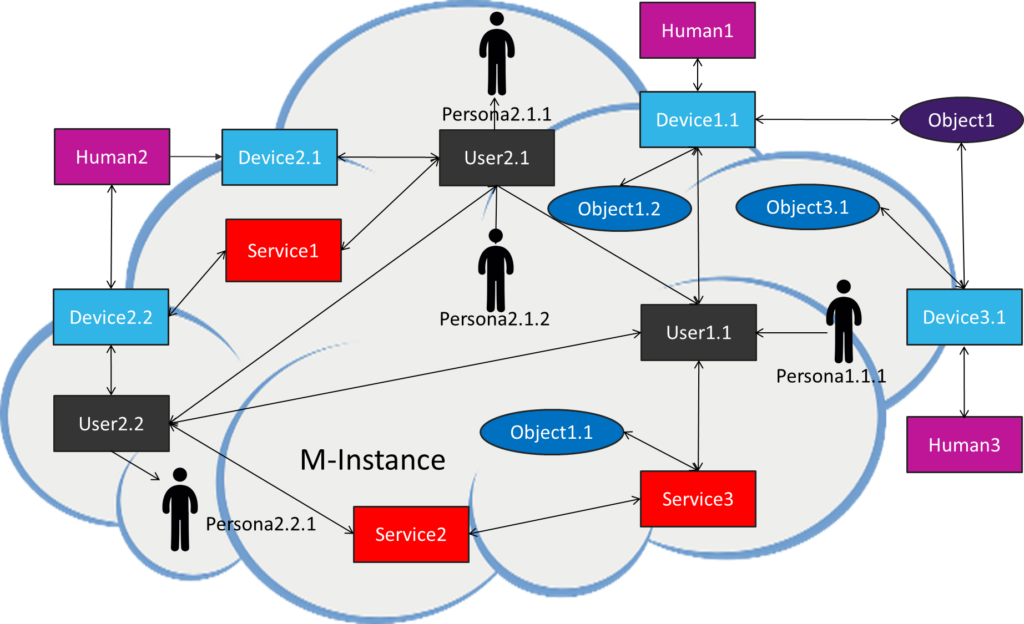
Figure 3 – M-Instance Model
In Figure 3 we see that:
- Human1 and Human3 are connected to the M-Instance via a Device, but Human2 is connected to the M-Instance with two Device.
- Human1 has deployed one User, User 2 two Users and Human3
- User1.1 of Human1 is rendered as one Persona1.1.1, User 2.1 of Human2 as two Personae (Persona2.1.1 and Persona2.1.2), and User2.2 as one Persona2.2.1.
- Object1 in the U-Environment is captured by 1 and Device3.1 and mapped as two distinct Objects: Object1.2 and Object3.1.
- Users and Services variously interact.
What are the interactions referred to in point 5. above? We assume that an M-Instance is populated by Services performing functions that are useful for the life of the M-Instance. We call standard functions “Actions”. MPAI has specified the functional requirements of a set of Actions:
- General Actions (Register, Change, Hide, Authenticate, Identify, Modify, Validate, Execute).
- Call a Service (Author, Discover, Inform, Interpret, Post, Transact, Convert, Resolve).
- M-Instance to M-Instance (MM-Add, MM-Animate, MM-Disable, MM-Embed, MM-Enable, MM-Send).
- M-Instance to U-Environment (MU-Actuate, MU-Render, MU-Send, Track).
- U-Environment to M-Instance (UM-Animate, UM-Capture, UM-Render, UM-Send).
The semantics of some of these Actions are:
- Identify: convert data and metadata into an Item bearing an Identifier.
- Discover: request a Service to provide Items and/or Processes with certain features.
- MM-Embed: place an Item at a particular M-Instance location (M-Location).
- MU-Render: select Items at an M-Location and render them at a U-Environment.
- UM-Animate: use a captured animation stream to animate a Persona.
How do interactions take place in the M-Instance?
A User may have the capability to perform certain Actions on certain Items but more commonly a User may ask a Device to do something for it, like capture an animation stream and use it to animate a Persona. The help of the Device may not be sufficient because MPAI assumes that an animation stream is not an Item until it gets Identified as such. Hence, the help of the Identify Service is also needed.
MPAI has defined the Inter-Process Communication Protocol (Figure 4) whereby
- A process creates, identifies and sends a Request-Action Item to the destination process.
- The receiving process
- May or may not perform the action requested
- Sends a Response-Action.
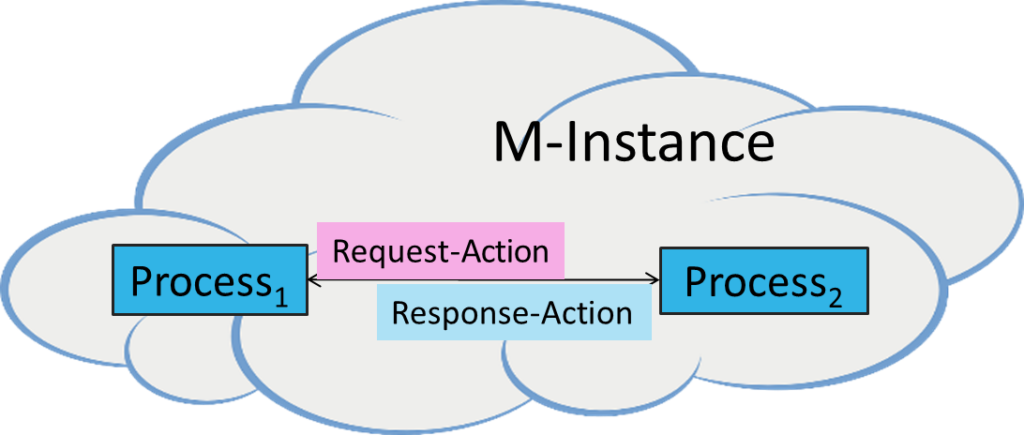
Figure 4 – The Inter-Process Interaction Protocol
Table 1 – The Inter-Process Interaction Protocol
Request-Action |
Response-Action |
Comments |
Request-Action ID |
Response-Action ID |
Unique ID |
Emission Time |
Emission Time |
Time of Issuance |
Source Process ID |
Source Process ID |
Requesting Process ID |
Destination Process ID |
Destination Process ID |
Requested Process ID |
Action |
|
The Action requested |
InItems |
OutItems |
In/Output Items of Action |
InLocations |
|
Locations of InItems |
OutLocations |
|
Locations of OutItems |
OutRights |
|
Expected Rights on OutItems |
The Request-Action payload includes the ID, the time, the requesting process and destination process IDs, the Action requested, the InItems on which the action is applied, where the InItems are found and the resulting OutItems are found, and the Rights the requesting process needs to have in order to act on the OutItems.
Having defined standard Actions, here is how standard Items are defined:
- General (M-Instance, M-Capabilities, M-Environment, Identifier, Rules, Rights, Program, Contract)
- Human/User-related (Account, Activity Data, Personal Profile, Social Graph, User Data).
- Process Interaction (Message, P-Capabilities, Request-Action, Response-Action).
- Service Access (AuthenticateIn, AuthenticateOut, DiscoverIn, DiscoverOut, InformIn, InformOut, InterpretIn, InterpretOut).
- Finance-related (Asset, Ledger, Provenance, Transaction, Value, Wallet).
- Perception-related (Event, Experience, Interaction, Map, Model, Object, Scene, Stream, Summary).
- Space-related (M-Location, U-Location).
Here are a few examples of the Item semantics:
- Rights: the Item describes the ability of a process to perform an Action on an Item at a time and at M-Location.
- Social Graph: the log of a process, e.g., a User.
- P-Capabilities: the Item describes the Rights held by a process and related abilities.
- DiscoverIn: the description of the User’s request.
- Asset: an Item that can be transacted.
- Model: data exposing animation interfaces.
- M–Location: delimits a space in the M-Instance.
We also need to define several entities – called Data Types – used in the M-Instance:
- Location and time (Address, Coordinates, Orientation, Point of View, Position, Spatial Attitude, Time).
- Transaction-related (Amount, Currency).
- Internal state of a User (Cognitive State, Emotion, Social Attitude, Personal Status).
Finally, we need to address the issue of a process in M-InstanceA requesting a process in another M-InstanceB to perform Actions on Items. In general, it is not possible for a process in M-InstanceA to communicate with a process in M-InstanceB because of security concerns, but also because the other M-InstanceB may use different data types. MPAI solves this process by extending the Inter-Process Interaction Protocol and introducing two Services:
- Resolution ServiceA: can talk to Resolution ServiceB.
- Conversion Service: can convert the format of M-InstanceA data into the format of M-InstanceB.
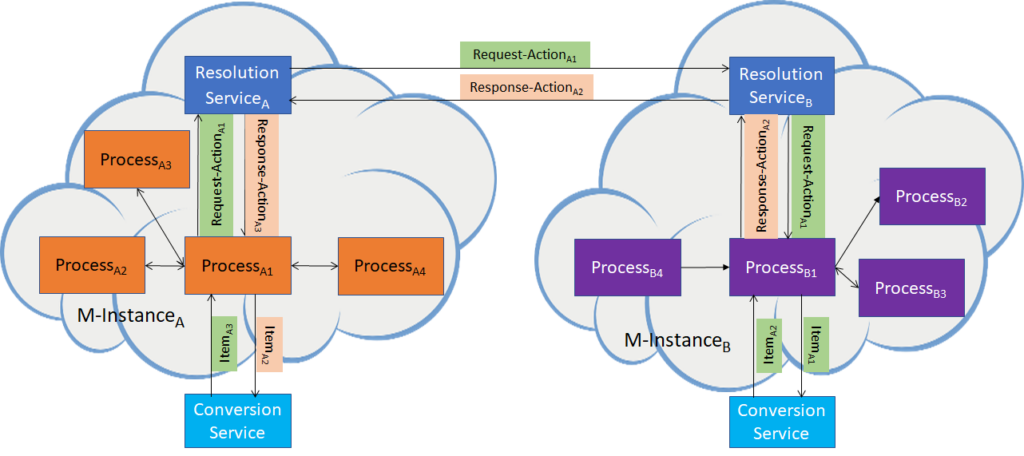
Figure 5 – The Inter-Process Interaction Protocol between M-Instances
This is a very high-level description of the MPAI Metaverse Model – Architecture standard that enables Interoperability of two or more M-Instances if they:
- Rely on the Operation Model, and
- Use the same Profile Architecture, and
- Either the same technologies, or
- Independent technologies while accessing Conversion Services that losslessly transform Data of an M-InstanceA to Data of an M-InstanceB.