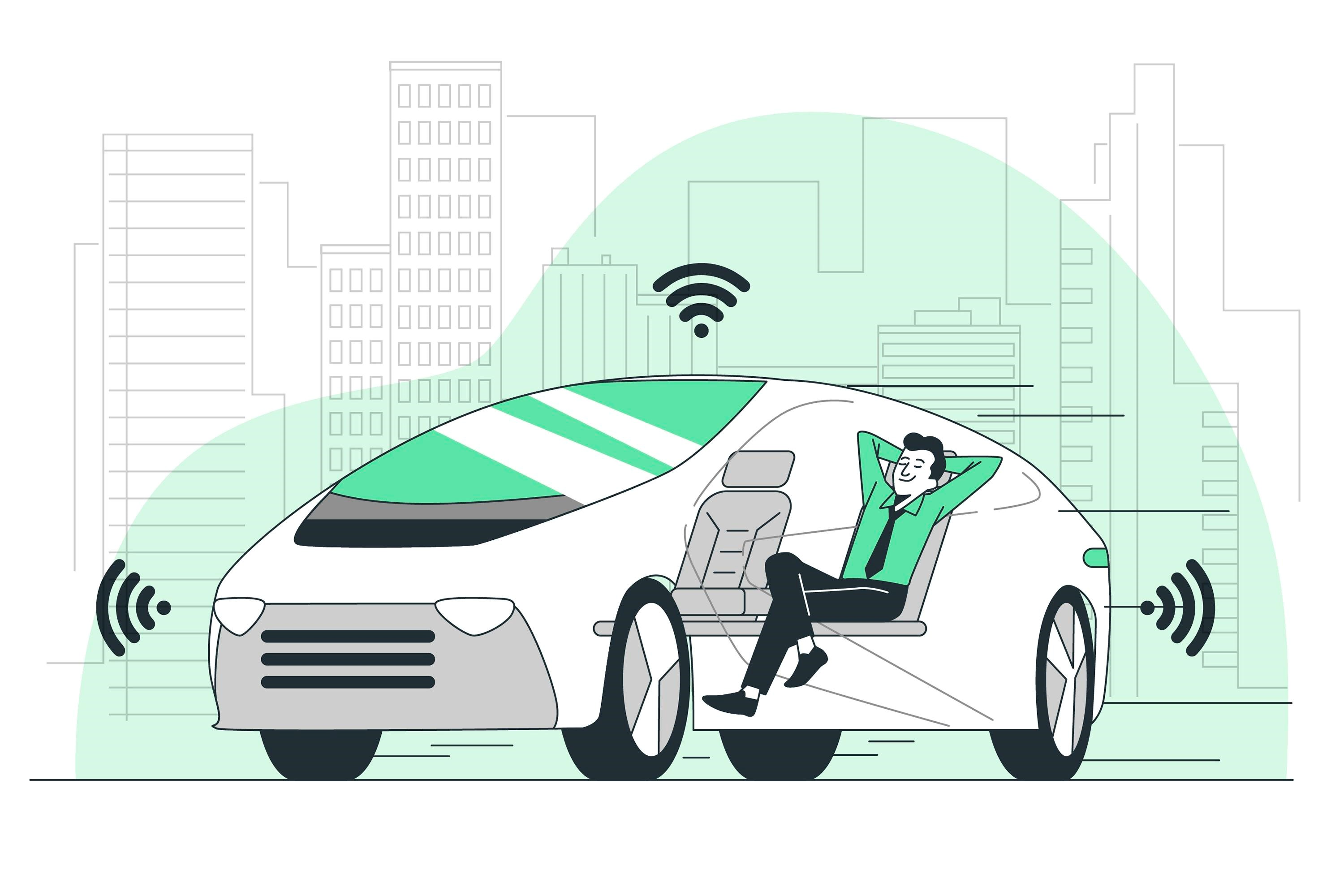
An overview of the Connected Autonomous Vehicle – Technologies (CAV-TEC) standard
1. Introduction
The Connected Autonomous Vehicle (CAV) specified by CAV-TEC is a system that instructs a vehicle with at least three wheels to reach a Destination from a current Pose at the request of a human or a process. It does so by exploiting information captured and processed by the vehicle and communicated by other CAVs while respecting the local traffic law, Figure 1 represents an example of the type of environment that a CAV is requested to traverse and Figure 2 depicts the four subsystems of which a CAV is composed, although this partitioning is not a functional requirement as components of a subsystem may be located in another subsystem, provided the interfaces specified by CAV-TEC are preserved.
Figure 1 – An example of an environment traversed by a CAV
In Figure 2, a human approaches a CAV and requests the Human-CAV Interaction Subsystem (HCI) to be taken to a destination using a combination of four media – Text, Speech, Face, and Gesture. Alternatively, a remote process may make a similar request to the CAV.
Figure 2 – The subsystems of a CAV
Either request is passed to the Autonomous Motion Subsystem (AMS), which requests the Environment Sensing Subsystem (ESS) to provide the current CAV Pose. With current Pose information, the Destination, the AMS can propose one or more Routes by accessing Offline Maps. Eventually the human or process can select a Route.
With the human aboard, the AMS continues to receive environment information from the ESS – possibly complemented by information received from other CAVs in range – and instructs the Motion Actuation Subsystem to make appropriate motions.
2. Human-CAV Interaction
The CAV-HCI Reference Model of Figure 3 explains the operation of the HCI in its interaction with humans.
Figure 3 – Reference Model of CAV-HCI
The Audio-Visual Scene Description (AVS) monitors the environment and produces Audio-Visual Scene Descriptors from which it extracts Speech Scene Descriptors and from these, Speech Objects corresponding to any speaking humans in the environment surrounding the CAV. Visual Scene Descriptors may also be extracted in the form of Face and Body Descriptors of all humans present.
The CAV activates Automatic Speech Recognition (ASR) to have the speech of each human recognised and converted into Recognised Text. Each Speech Object is identified according to their position in space. The CAV also activates the Visual Object Identification (VOI) that is able to produce the Instance IDs of Visual Objects as indicated by humans.
Natural Language Understanding (NLU) processes the Speech Objects, produces Refined Text, and extracts Meaning from the Text of each input Speech. This process is facilitated by the use of the IDs of the Visual Objects provided by VOI.
Speaker Identity Recognition (SIR) and Face Identity Recognition (FIR) help the CAV to reliably obtain the Identifiers of the the humans the HCI is interacting with. If the Face ID(s) provided by FIR correspond to the ID(s) provided by SIR, the CAV may proceed to attend to further requests. Especially with humans aboard, Personal Status Extraction (PSE) provides useful information regarding the humans’ state of mind by extracting their Personal Status.
The CAV interacts with humans through Entity Dialogue Processing (EDP). When a human requests to be taken to a Destination, the EDP interprets and communicates the request to the Autonomous Motion Subsystem (AMS). A dialogue may then ensue where the AMS may offer different choices to satisfy potentially different human needs (e.g., a long but comfortable Route or short but less predictable).
Then, while the CAV moves to the Destination, the HCI may have a conversation with the humans, show the Full Environment Descriptors developed by the AMS to the passengers, and may communicate information about the CAV from the Ego AMS or more generally from the HCIs of remote CAVs.
The HCI responds using the two main outputs of the EDP: Text and Personal Status. These are used by the Personal Status Display (PSD) to produce the Portable Avatar of the HCI conveying Speech, Face, and Gesture synthesised to render the HCI Text and Personal Status. Audio-Visual Scene Rendering (AVR) renders Audio, Speech, and Visual information using the HCI Portable Avatar. Alternatively, it can display the AMS’s Full Environment Descriptors from the Point of View selected by the human.
The HCI interacts with passengers in several ways:
- By responding to commands/queries from one or more humans at the same time, e.g.:
- Commands to go to a waypoint, park at a place, etc.
- Commands with an effect in the cabin, e.g., turn off air conditioning, turn on the radio, call a person, open a window or door, search for information, etc.
- By conversing with and responding to questions from one or more humans at the same time about travel-related issues, e.g.:
- Humans request information, e.g., time to destination, route conditions, weather at destination, etc.
- Humans ask questions about objects in the cabin.
- By following the conversation on travel matters held by humans in the cabin if
- The passengers allow the HCI to do so, and
- The processing is carried out privately inside the CAV.
3. Environment Sensing Subsystem
The operation of the Environment Sensing Subsystem (ESS) is best explained using the Reference Model of the CAV-ESS subsystem depicted in Figure 4.
Figure 4 – Reference Model of CAV-ESS
When the CAV is activated in response to a request by a human owner or renter or by a process, Spatial Attitude Generation continuously computes the CAV’s Spatial Attitude relying on the initial Motion Actuation Subsystem’s Spatial Attitude, and information from the Global Navigation Satellite Systems (GNSS), if available.
An ESS may be equipped with a variety of Environment Sensing Technologies (EST). CAV-TEC assumes they are (but not required to all be supported by an ESS implementation) Audio, LiDAR, RADAR, Ultrasound, and Visual. Offline Map is considered as an EST.
An EST-specific Scene Description receives EST-specific Data Objects, produces EST specific Scene Descriptors which are integrated into the Basic Environment Descriptors (BED) by the Basic Environment Description using all available sensing technologies, Weather Data, Road State, and possibly the Full Environment Descriptors of previous instants provided by the AMS. Note that, although in Figure 4 each sensing technology is processed by an individual EST, an implementation may combine two or more Scene Description AIMs to handle two or more ESTs, provided the relevant interfaces are preserved. An EST-specific Scene Description may need to access the BED of previous instants and may produce Alerts that are immediately communicated to AMS.
The Objects in the BEDs may carry Annotations specifically related to traffic signalling, e.g.: Position and Orientation of traffic signals in the environment, Traffic Policemen, Road signs (lanes, turn right/left on the road, one way, stop signs, words painted on the road), Traffic signs – vertical signalisation (signs above the road, signs on objects, poles with signs), Traffic lights, Walkways, and Traffic sounds (siren, whistle, horn).
4. Autonomous Motion Subsystem
The Reference Model of the CAV-AMS subsystem depicted in Figure 5 explains the operation of the Autonomous Motion Subsystem (AMS) is.
Figure 5 – Reference Model of CAV-AMS
When the HCI sends the AMS a human or process request to move the CAV to a Destination, Route Planning uses the Basic Scene Descriptors from the ESS and produces a set of Waypoints starting from the current Pose up to the Destination.
When the CAV is in motion, Route Planning causes Path Selection Planning to generate a set of Poses to reach the next Waypoint. Full Environment Description may request the AMSs of Remote CAVs to send (subsets of) their Scene Descriptors and integrates all sources of Environment Descriptors into its Full Environment Descriptors (FED), and may also respond to similar requests from Remote CAVs.
Motion Selection Planning generates a Trajectory to reach the next Pose in each Path. Traffic Obstacle Avoidance receives the Trajectory and checks if any Alert was received that would cause a collision with the current Trajectory. If a potential collision is detected, Traffic Obstacle Avoidance requests a new Trajectory from Motion Planner, otherwise Traffic Obstacle Avoidance issues an AMS-MAS Message to Motion Actuation Subsystem (MAS).
The MAS sends an AMS-MAS Message to AMS informing it about the execution of the AMS-MAS Message received. The AMS, based on the received AMS-MAS Messages, may discontinue the execution of the earlier AMS-MAS Message, issue a new AMS-MAS Message, and inform Traffic Obstacle Avoidance. The decision of each element of the chain may be recorded in the AMS Memory (“black box”).
5. Motion Actuation Subsystem
The operation of the (MAS) is explained using the Reference Model of the CAV-MAS subsystem depicted in Figure 6.
Figure 6 – Reference Model of CAV-AMS
When the AMS Message Interpretation receives the AMS-MAS Message from the AMS, it interprets the Messages, partitions it into commands, and sends them to the Brake, Motor, and Wheel mechanical subsystems. CAV-TEC is silent on how the three mechanical subsystems process the commands but specifies the format of the commands issued to AMS Message Interpretation. The result of the interpretation is sent as an AMS-MAS Message to AMS.
MAS includes two more AIMs. Spatial Attitude Generation computes the initial Ego CAV’s Spatial Attitude using the Spatial Data provided by Odometer, Speedometer, Accelerometer, and Inclinometer. This initial Spatial Attitude is sent to the ESS to integrate its GSNN-based Spatial Attitude. Ice Condition Analysis augments the Weather Data by analysing the Brake, Motor, and Wheel mechanical subsystems’ responses and sends the augmented Weather Data to the ESS.
6. Conclusions
CAVs promise to bring benefits that will positively affect industry, society, and the environment, for example:
- Saving lives and reducing injuries by removing human error thanks to a machine less prone to errors.
- Giving humans more time for rewarding activities, such as interpersonal communication.
- Optimising the use of vehicles and infrastructure.
- Reducing congestion and pollution.
- Supporting elderly and disabled people.
Therefore, society and individuals will be positively impacted by the transformation of today’s “niche market” into tomorrow’s vibrant “mass market” of Connected Autonomous Vehicles. A market of interchangeable components conforming with the CAV-TEC V2.0 specifications can offer affordable and safe Connected Autonomous Vehicles sooner and more efficiently than waiting for market forces to produce “monolithic” cars with progressively higher SAE Levels.
The CAV-TEC Reference Model can create a competitive market offering increasingly performing components until a new, more powerful reference model will eventually replace the model with another, initiating a new sequence of performance improvements. The Reference Model will help:
- Researchers to optimise component technologies.
- Component manufacturers to bring their standard-conforming components to market once they are mature.
- Car manufacturers to access an open global market of interchangeable components.
- Regulators to oversee conformance testing of components following standard procedures.
- Users to rely on Connected Autonomous Vehicles whose operation they can explain to a large extent.