If media have become so pervasive, it is because smart use of data processing has reduced the amount of data generated by audio and video. Digital media can give more, but the spirit that has produced MP3, digital television, audio and video on the internet, DASH and so much more has waned
The new organisation MPAI – Moving pictures, audio and data coding by Artificial Intelligence (AI) – has the necessary propulsive thrust. MPAI AI as the enabling technology to code data.
The term AI is in the MPAI title because AI is the enabling technology extending coding from compression (i.e., less bits for a similar result) to understanding (i.e., what the bits mean), and the use of coding from media, to many more data types.
Data processing remains a valid alternative to A-I in many domains, though…
MPAI has defined 5 pillars on which it bases its operation. The formulation of the process benefits from 30+ years of standardisation where a huge organisation – MPEG – was created from nothing and processes have been fine tuned from day-to-day real-world experience.
Pillar #1 – the process
MPAI likes to call itself, as the domain extension in mpai.community implies, a “community”. The development of MPAI standards is divided in 4 phases: Preparation, Framework Licence, Standard Development and Standard approval. They are characterised by those who are allowed to participate in each phase. In total there are 7 stages, as can be seen from the Figure 1
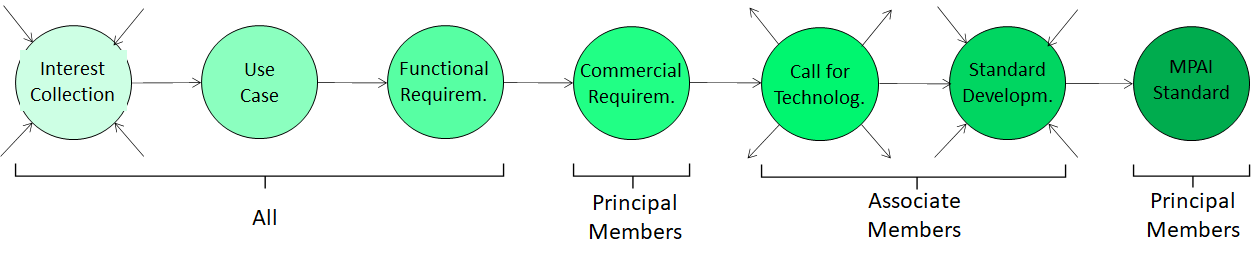
Figure 1 – The MPAI standard development stages
Phase 1 – Preparation.
Stage 0 – Interest Collection (IC): Members as well as non-members may submit proposals. These are collected and harmonised. Some proposals get merged with other similar proposals. Some get split because the harmonisation process so demands. The goal is to identify proposals of standard that reflect the proponent’s wishes while making sense in terms of specification and use across different environments.
Stage 1 – Use Case (UC): Use Cases are full characterised and description of the work program that will produce the Functional Requirements is developed.
Stage 2 – Functional Requirements (FR): detailed functional requirements of the Use Case are developed.
In the three stages above, MPAI is “open” in the sense that anybody interested may participate. However, if an MPAI Member wants to discuss a confidential proposal, only MPAI members may attend. From the Commercial Requirements stage onward, non-members are not allowed to participate (but they may become members at any time).
Phase 2 – Framework Licence
Stage 3 – Commercial Requirements (CR): in a supply contract the characteristics (Functional Requirements) and the conditions (Commercial Requirements) are described. Antitrust laws do not permits that sellers (technology providers) and buyers (standard users) sit together and agree on values such as numbers, percentage or dates. However, sellers (technology providers) may indicate supply conditions, without values. Therefore, the embodiment of the Commercial Requirements, i.e. the Framework Licence, will not contain such details. Only Principal Members who declare they will make technical contributions to the standard can participate in the drafting of the Framework Licence.
Phase 3 – Standard Development
Stage 4 – Call for Technologies (CT): Once both Requirements are available, MPAI is in a position to draft the CfT. Anybody may respond to a CfT. However, if one of their proposed technologies from a non-member is accepted, the responder must join MPAI.
Stage 5 – Standard Development (SD): the Development Committee in charge reviews the responses and develops the standards.
Phase 4 – Standard approval
Stage 6 – MPAI Standard (MS): Only Principal Members may vote to approve the standard, hence trigger its publication. Associate Members, however, may become Principal Members at any time.
For each standard project, transition to each of the 7 stages of Figure 1 must approved by a resolution of the General Assembly.
Pillar #2 & #3 – AI Modules and Framework
MPAI makes assumptions about the internal structure of an AI system to provide levels of guarantee about the “ethical performance” of an AI system that implements an MPAI standard.
- An implemention of an MPAI-specified Use Case is subdivided into functional components called AI Modules (AIM) that use Artificial Intelligence (AI) or Machine Learning (ML) or traditional Data Processing (DP) or a combination of these and are implemented in software or hardware or mixed hardware and software.
- An AI system implementing a Use Case is an aggregation of AIMs, specified by the Use Case, in a topology specified in the standard, interconnected as topology specified and executed inside an AI Framework (AIF).
The 2 basic elements of the MPAI standardisation are represented in Figure 42 e 9and Figure 3.
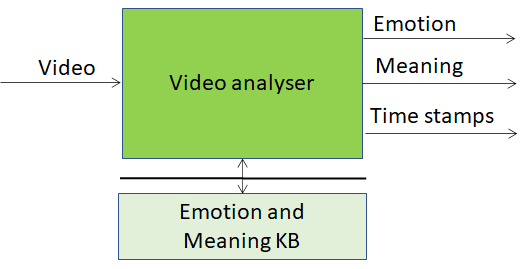 |
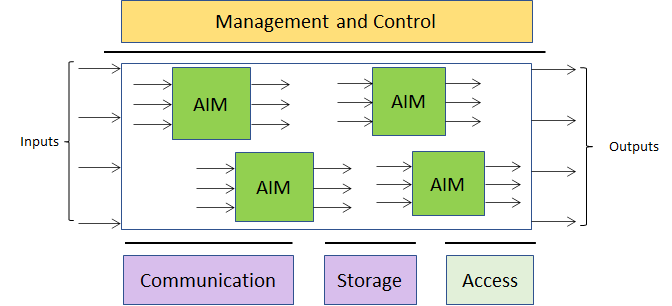 |
Figure 49 – The MPAI AI Module (AIM) |
Figure 50 – The MPAI AI Framework (AIF) |
Figure 2 depicts a video coming from a camera shooting a human face. The function of the AIM (green block) is to detect the emotion on the face and the meaning of the sentence the human is uttering. The AIM can be implemented with a neural network or with DP technologies. In the latter case, the AIM accesses a knowledge base external to the AIM.
The input data enter the Execution area of the AIF (Figure 3) where the workflow is executed under the supervision of Management and Control. AIMs communicate via the AIF’s Communication and Storage infrastructure and may access static or slowly changing data sources (e.g., those of Figure 2) called Access. The result of the execution of the workflow is provided as output data.
Pillar #4 – IPR Guidelines
Seventy years ago, when ISO was established, it made sense to ask a participant in the standardisation process to make a declaration of availability to licence their technology at fair and reasonable terms and non discriminatory conditions (FRAND). Any IP item was typically held by one company. Actually that company most likely was approaching ISO because it had already products on the market, was already licensing its technology and just wanted ISO to ratify the status quo.
Forty years later, when MPEG started releasing its standards, the situation was entirely different. Each participant had IP but most participants were interested in using the standard.
Another 20 years later, the situation had changed beyond recognition. Each participant had IP, but most participant were not interested in using the standard, only in monetising their IP.
MPAI fully endorses IP as the engine of progress but cautions against standards released with FRAND promises. MPAI has not washed its hands of the IP issue, but has developed the notion of framework Licence. This is the IPR holders’ business model adopeted to monetise their IP in a standard without values: $, %, dates etc. This is the practice:
- Before the standard is developed: Active members develop & adopt the Framework Licence
- While the standard is developed: Members declare to make available their licences according to the Framework Licence after the standard is approved for any submissions they make.
- After the standard is developed: All members declare they will get a licence for other members’ IPRs, if used, within 1 year after publication of IPR holders’ licensing terms.
Non members must get a licence from IPR holders to use an MPAI standard.
You can see an example of actual Framework Licence.
Pillar #5 – Ethical AI
MPAI is not alone in being aware of the impact AI will have on humans and society. Instead of just raising concerns about bias in AI, MPAI intends to offer practical solutions.
As an example, MPAI’s AIM-AIF approach is already capable to enhance explainability of MPAI standards’ implementations. By Explainability we mean “the ability to trace the output of an AI system back to the inputs that have produced it”.
The solution MPAI is working on will provide the means to test the Performance of MPAI standard implementations. An element of the solution is an identification system that helps users check whether an AI system is a tested implementation of an MPAI standard.
Who benefits from the MPAI approach?
- Component providers can offer conforming AIMs to an open competitive market and test or have them tested for performance.
- Application developers wishing to develop complex components may have some but not all AIMs they need. However, they can find the AIMs they need on the open competitive market.
- Consumers have a wider choice of better AI applications from competing application developers. Theu can discriminate from generic AI systems from AI systems that implement AI standards,
- Innovation will be fuelled by the need for novel/more performing AIMs to face competition.
- Society can lift the veil of opacity from large and monolithic AI systems.
MPAI has been in operation since the 30th of September 2020. A few months after, it is developing, 4 standards and the Functional Requirements for 3 standards, and is honing 2 Use Cases. Figure 4 depcts the situation.
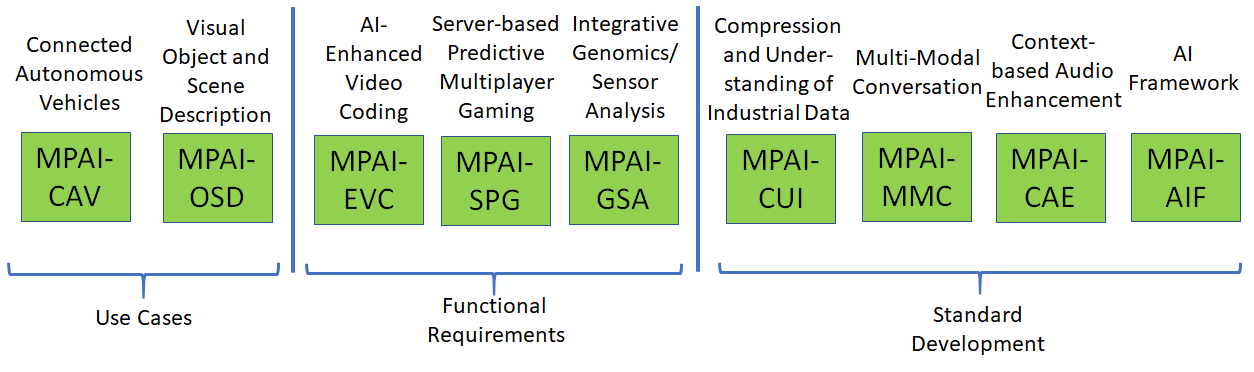
Figure 51 – Snapshot of the MPAI work plan